Computational Advances in Modern Neuroscience
Welcome to my blog! This summer, I am embarking on an exciting outreach adventure by sharing my PhD research with you. Each week, I will release a new blog post where I unpack a specific aspect of my scientific work. The best part? I will be presenting the information in bite-sized, easily understandable chunks of text! So, whether you are a fellow academic, a curious mind, or simply looking to expand your knowledge, this blog is here to serve you. Throughout the text and in each image you can find links to more detailed sources of information for the topics I discuss here. This week's blog post focusses on simulating brain areas with computational neuroscience. Let's get started!
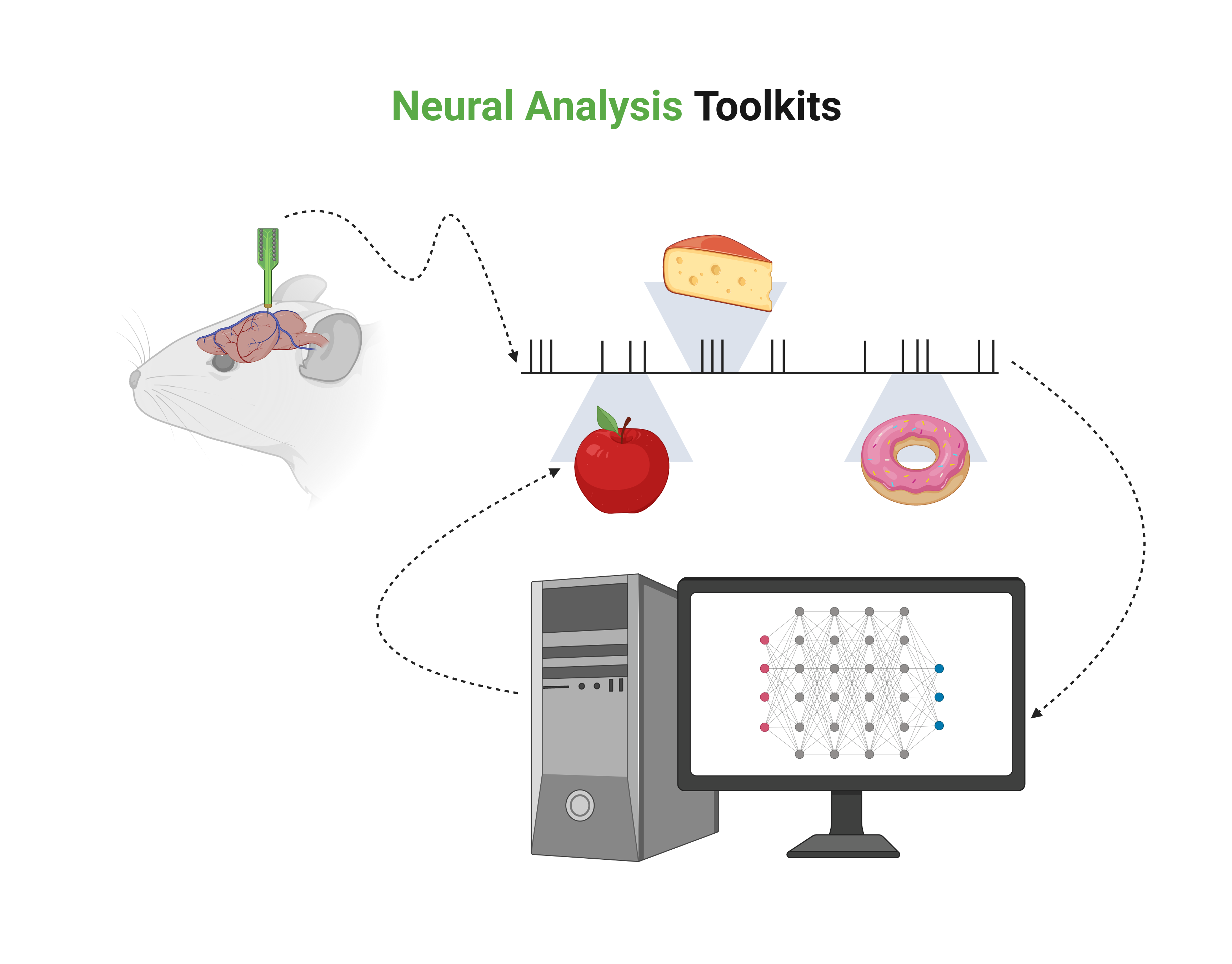
In addition to novel tool-kits developed to analyse the recent influx of experimental neuroscience data, such as deep-learned latent spaces for neural dynamics or topological data analysis based on persistent homology, computational neuroscience has also seen the rapid development of increasingly larger simulations of networks of model neurons. While computationally expensive, large-scale computer models of networks of neurons form an important connection between single neuron and system-level analysis of the brain. Being able to tune various parameters of neuron models, based on experimental inputs, is a way to use computational models as a counterpart to laboratory experiments. These network models represent neurons at various levels of abstraction, with three common tiers: biophysically-detailed models, point-neuron models, and population-level models.
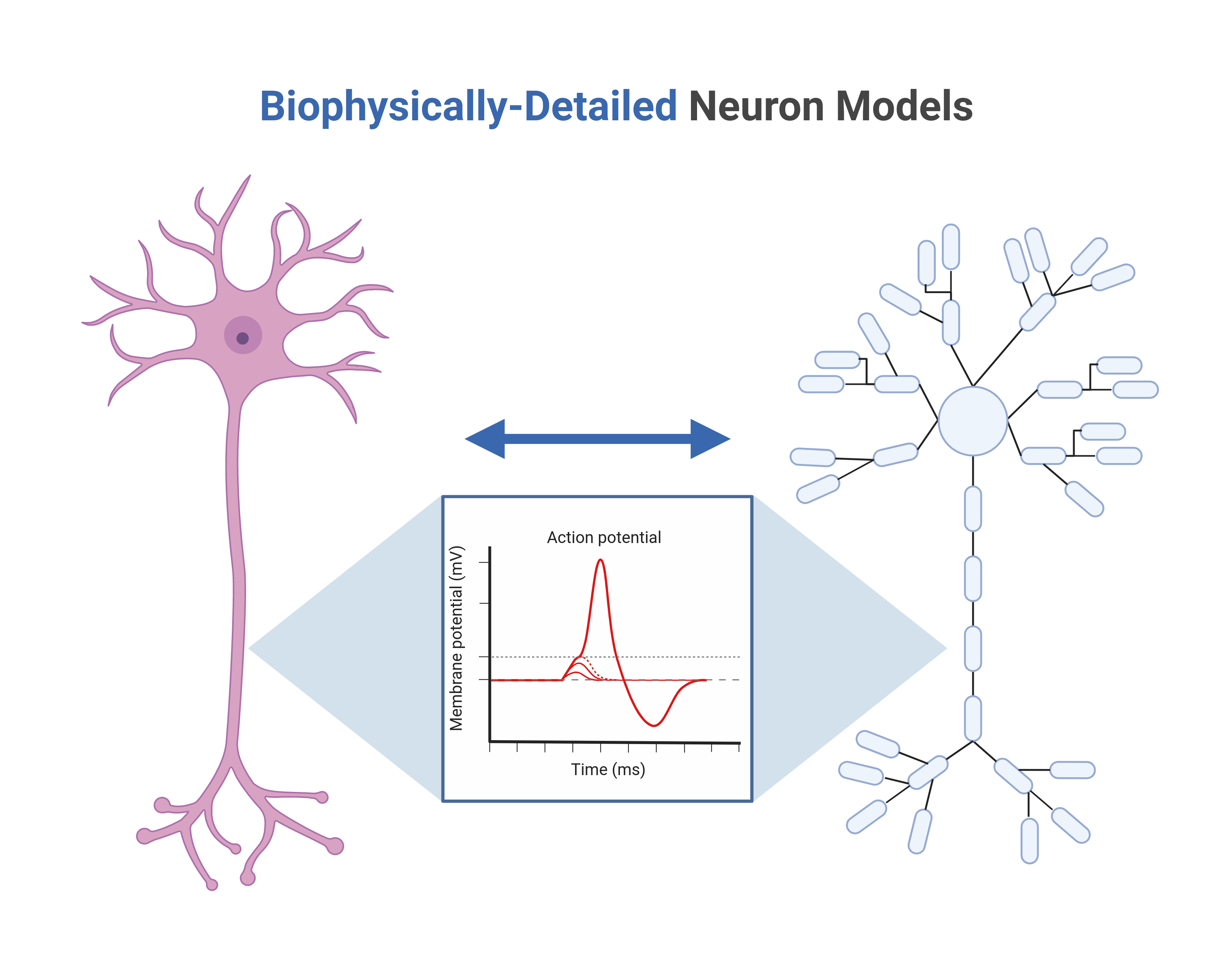
Biophysically-detailed models dive deep into the intricacies of single neurons, replicating their physical morphology through compartmentalized representations. Point-neuron models simplify the spatial aspect and rely on integrate-and-fire dynamics to convert synaptic inputs into output signals. Population-level models, on the other hand, employ a single population-density equation to capture the voltage distribution of a neuron population. Recent breakthroughs have even seen biophysically-detailed models being used to reconstruct the microcircuitry of brain regions, like the somatosensory cortex in juvenile rats and the primary visual cortex in mice, all based on limited biological data. To ensure the accuracy of these biophysically-detailed network models, simulations undergo rigorous comparisons with experimental data, including voltage traces and spiking statistics.
 Recording.png)
Moreover, reconstructed microcircuitry of brain regions are scrutinized against population-level metrics such as local field potentials (LFPs), electrocorticography (ECoG), and electroencephalography (EEG) measurements. LFPs capture the low-frequency electric potentials within gray matter, and these signals, combined with other data sources, help researchers pinpoint critical parameters and network geometries in brain simulations. Importantly, these models are not just about understanding known data; they can also predict ECoG and EEG signals based on underlying neural activity. For instance, they can forecast how different genetic mutations - such as those found in severe mental disorders - might impact EEG measurements, offering new avenues for matching genetic information with clinical biomarkers of severe mental disorders.
The content of this blog post reflects my personal opinions and insights and should not be attributed to my employer or investors. The information provided in this post is for educational purposes only and should not be construed as medical advice. It is crucial to consult with medical professionals for any mental or physical healthcare concerns. All images featured in this blog post were created using Biorender.com under an academic license. These blog posts are derived from excerpts of my PhD thesis, based on research conducted at the University of Oslo, which you can also read on this website.