Distilling Computational Neuroscience
Welcome to my blog! This summer, I am embarking on an exciting outreach adventure by sharing my PhD research with you. Each week, I will release a new blog post where I unpack a specific aspect of my scientific work. The best part? I will be presenting the information in bite-sized, easily understandable chunks of text! So, whether you are a fellow academic, a curious mind, or simply looking to expand your knowledge, this blog is here to serve you. Throughout the text and in each image you can find links to more detailed sources of information for the topics I discuss here. This week's blog post focusses on using deep learning to accelerate computational neuroscience. Let's get started!
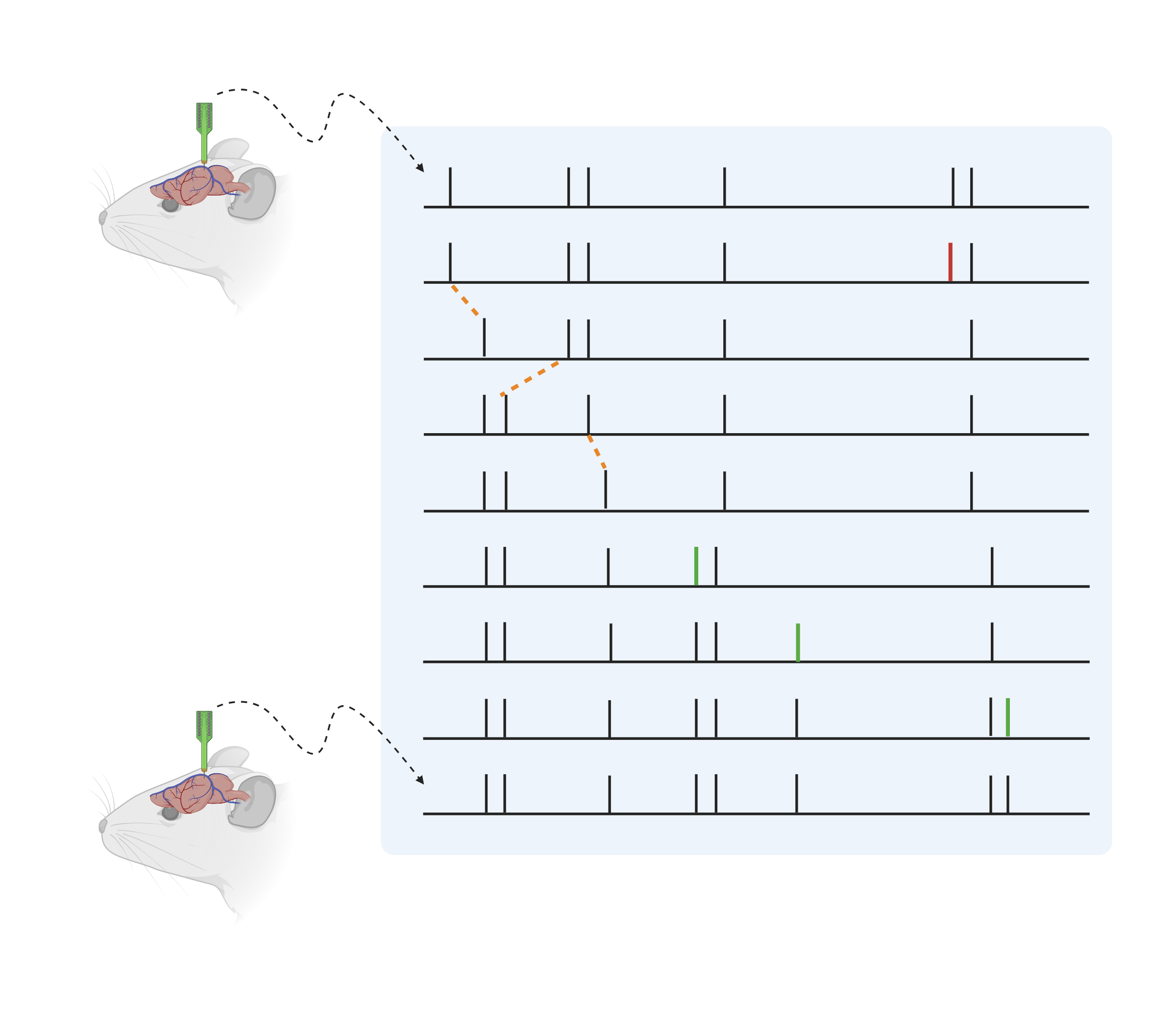
Despite decades of research investigating the brain through molecular, cellular, and animal studies, the complex principles governing the brain remain mostly unknown. Computational neuroscientists simulate and analyze neuronal data to make progress in understanding interactions between neurons. However, as discussed in the previous blog post, to simulate or analyze signals in entire brain areas, large amounts of computational resources are required. For instance, simulating a few seconds of a part of a biologically realistic model of the mouse primary visual cortex requires a large supercomputer. Similarly, topological data analysis of neuronal spike train data is limited by the computational cost of synchronicity calculations.
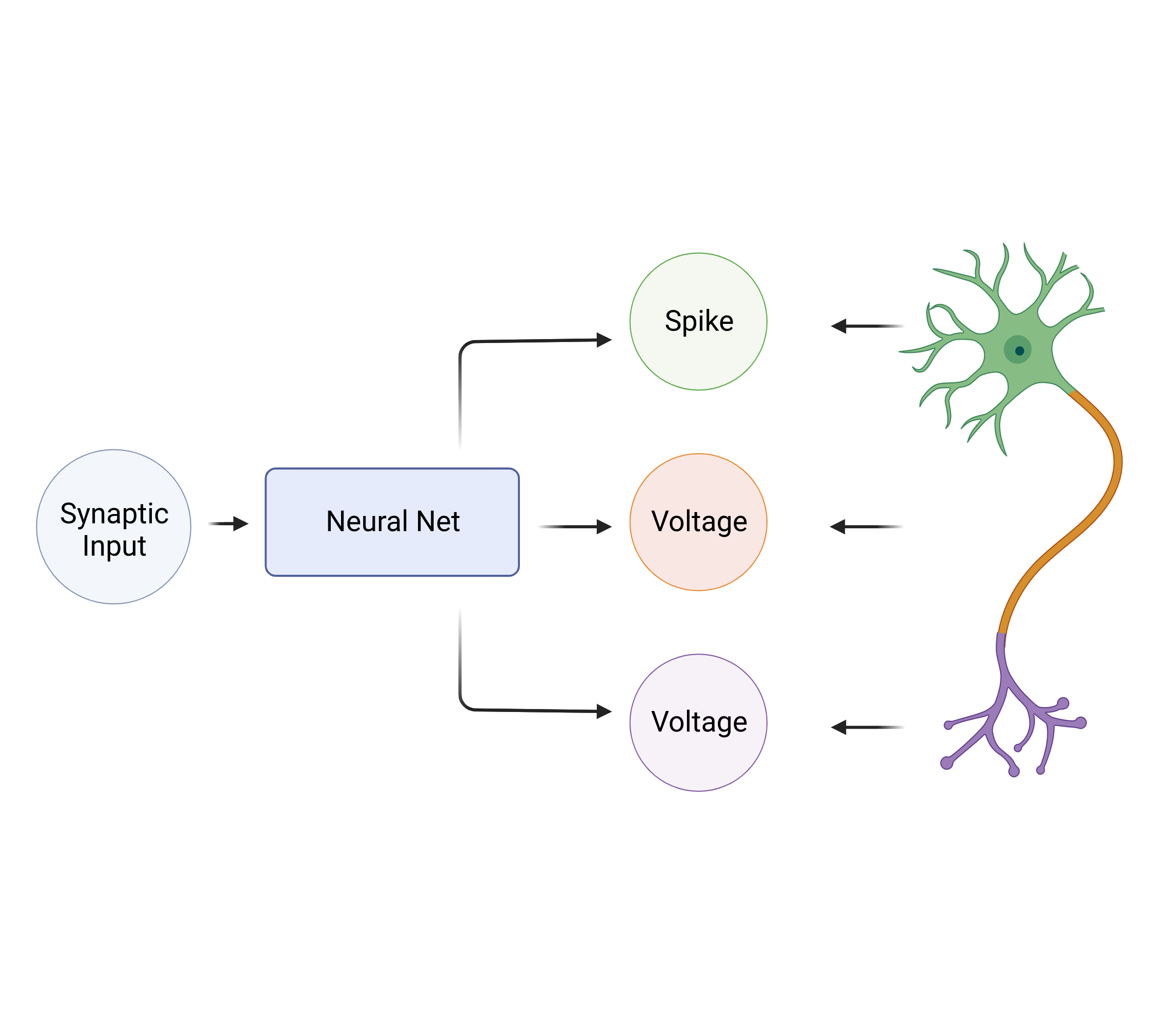
Computational resources required by biophysically detailed neuron models are not always available outside large academic institutions, and the large runtime limits flexible use of these models. Recent developments, including GPU-based solutions and simplified neuron simulations, have begun to address these challenges but to be able to run even larger and faster simulations, recent attention has turned to the idea of distilling computationally intensive biophysically-detailed neuron models into easier-to-evaluate artificial neural networks. These deep neural networks, relying on synaptic inputs and, in some cases, the previous time-step's membrane potential for each compartment, predict the generation or absence of an outgoing action potential or the membrane potential in the neuron's soma.
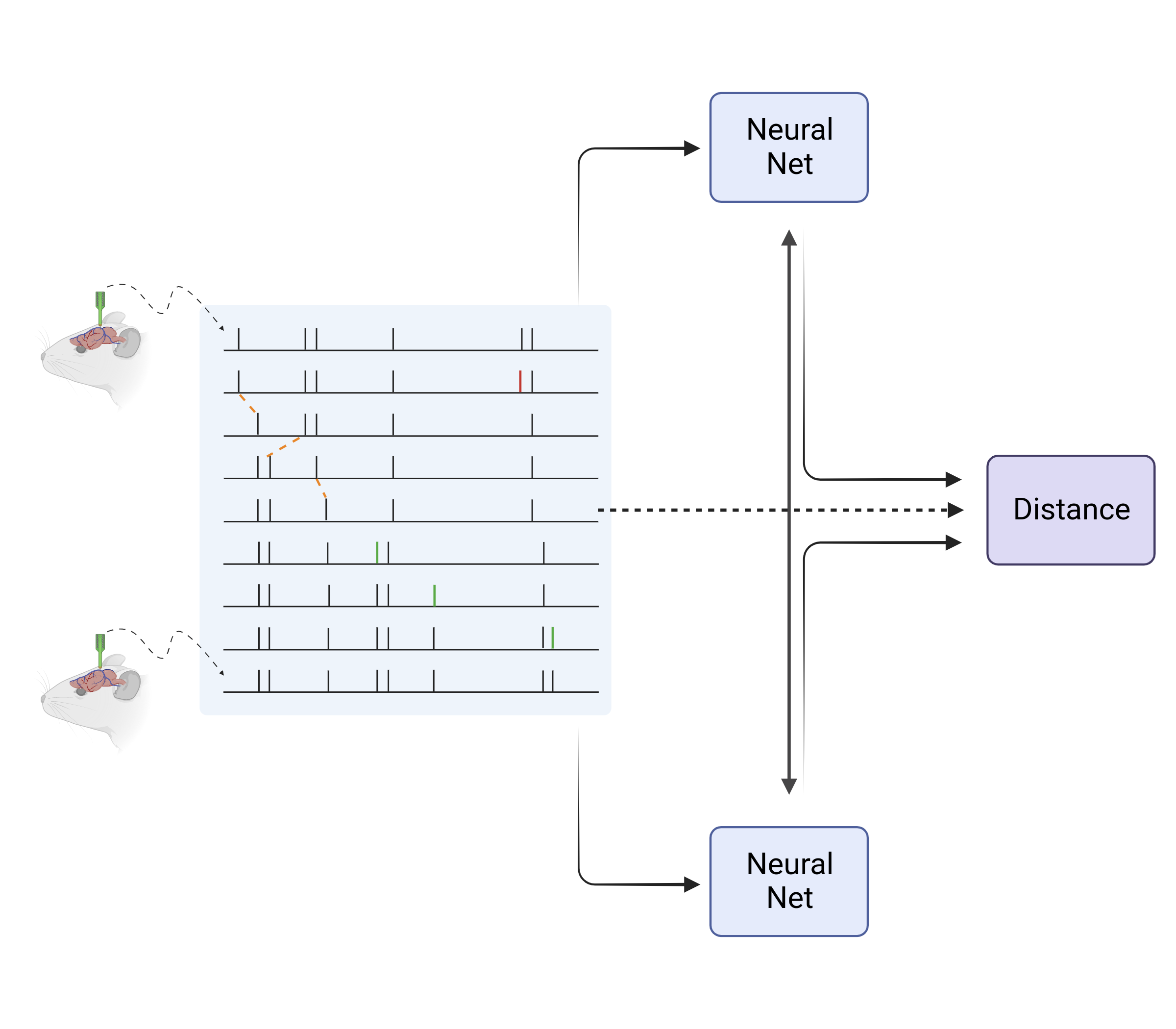
Spike synchronicity, plays a pivotal role in deciphering how groups of neurons communicate and coordinate their activities within the brain. The Victor-Purpura metric offers a quantitative measure of the degree of synchrony between spike trains, or sequences of action potentials, emitted by different neurons. Essentially, it helps us gauge how well neurons are "talking" to each other. However, calculating the Victor-Purpura metric can be computationally demanding, especially when dealing with vast datasets or complex neuronal networks. To address this challenge, we are aiming to harness the power of siamese neural networks, which are a type of deep learning architecture designed for comparing and measuring similarities between data points.
The content of this blog post reflects my personal opinions and insights and should not be attributed to my employer or investors. The information provided in this post is for educational purposes only and should not be construed as medical advice. It is crucial to consult with medical professionals for any mental or physical healthcare concerns. All images featured in this blog post were created using Biorender.com under an academic license. These blog posts are derived from excerpts of my PhD thesis, based on research conducted at the University of Oslo, which you can also read on this website.